A new report from big data and AI analytics acceleration platform SQream highlights widespread challenges across US enterprises, and shows why runaway costs may prove to be the biggest barrier standing in the way of AI adoption and data analytics.
AI’s slow march towards value
The tech industry has long promised that AI will usher in a new age of digital innovation, with advanced computer systems capable of being applied to a near-endless range of use cases, from producing persuasive prose, driving autonomous vehicles, managing factory floors and even helping to solve world hunger by optimizing food systems.
Yet while the automation and efficiency gains possible with AI have been on the radar for years, 2023 was when the technology’s adoption exploded thanks in part to the launch of OpenAI’s generative AI-driven applications.
This meant that 2024 was pegged to be the year that companies supporting AI-driven projects would finally begin to see some returns on their decision to invest, either in the form of increased revenue or improved profit margins thanks to this advanced technology.
However, deriving this much-awaited value still looks to be a point on the horizon. VCs poured $38.6 billion into AI and machine learning startups in the US in the first half of 2024, and it’s been reported that big tech leaders have been giving pep talks to quell fears that they’ve been caught up in a hype cycle that won’t deliver. Yet it’s not just investors, but also the thousands of companies with AI adoption projects already underway.
Part of the reason that this value is taking longer than expected to emerge can be traced back to running costs. From computer chips and data centers to cloud computing infrastructure, the component parts required to run big data analytics and AI projects come at a high price for companies.
Further, these costs increase exponentially along with the size of the datasets being crunched by the algorithms.
This week SQream, a data processing and analytic acceleration company based in New York, released the results of a landmark survey that looks at the latest analytics and AI trends across major US enterprise organizations.
Its report, titled “2024 State of Big Data Analytics: Constant Compromising Is Leading to Suboptimal Results”, lays bare just how widespread the issue of cost control is for major enterprises grappling with large, complex datasets, why this risks putting AI projects in jeopardy if not addressed, and what actions will help to remedy the situation.
‘Bill shock’ puts AI projects at risk
From intelligent product development, fraud detection, and cybersecurity to data-driven decision making and enhanced customer experiences, to name a few, it’s no surprise that organizations have been flocking towards AI and data analytic solutions to drive business growth, estimated at the equivalent of a staggering $2.6 trillion to $4.4 trillion annually.
However, SQream’s groundbreaking report reveals that, in reality, these gains are proving extremely costly to achieve. The insights in the report are based on a survey that included 300 senior data management professionals from US companies with at least $5M+ annual spend on cloud and infrastructure.
Despite the generous amount of budget already allocated to this area of business operations, costs continue to rise. 71% of respondents – that’s more than 2 out of 3 companies – reported they are surprised by the high costs of their cloud analytics bill.
Meanwhile, a shocking 98% of respondents had experienced project failure in 2023.
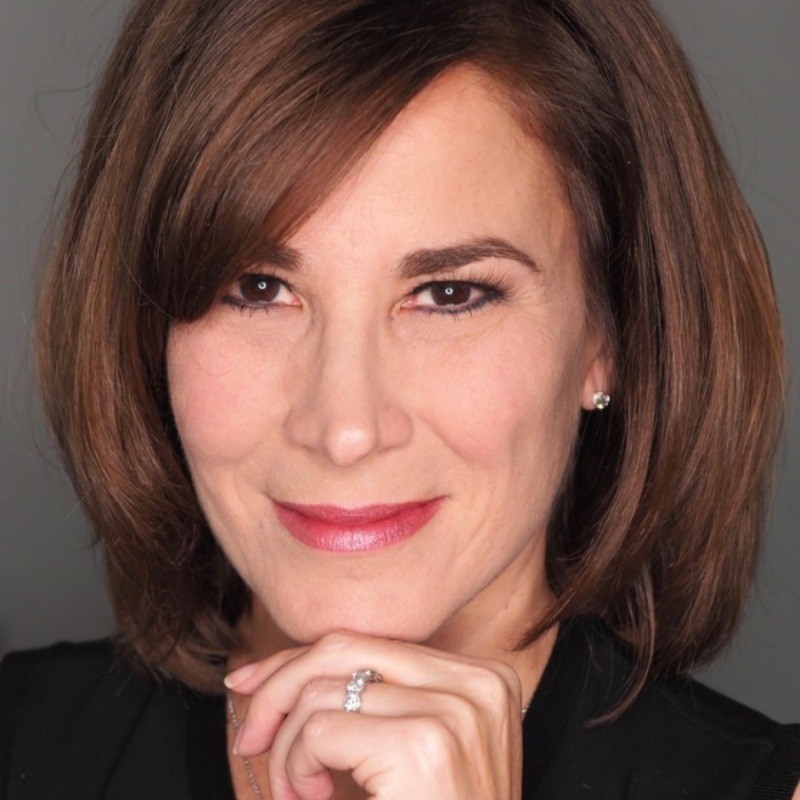
Deborah Leff, Chief Revenue Officer of SQream, commented in the company’s press release: “This survey underscores the widespread nature of these data management challenges for large enterprises.”
As more systems move to the cloud, operational processes are now largely digitized, and enterprise organizations are left trying to handle immense data sets that are increasingly costly to store and maintain. Further, individual AI queries become more expensive due to the amount of computational power needed to process these mega data volumes.
As a result, many leaders reported that their AI projects are often compromised as a result. The top contributing factor to project failures in 2023 was insufficient budget (29%). Meanwhile, 41% of companies consider the high costs involved in ML experimentation to be the primary challenge they face.
Here lies the crux of the real barrier to AI adoption which enterprises must overcome in order to make these projects sustainable to run.
Matan Libis, VP Product at SQream, explained further; “To get ahead in the competitive future of AI, enterprises need to ensure that more big data projects reach the finish line. Constant compromising, including on the size of data sets and complexity of queries, is a huge risk factor that corporate leaders need to address in order to effectively deliver on strategic goals.”
A shift in data management strategy
Up until now, the go-to method to add more power to big data projects was to add more CPUs. Yet, according to the report, this approach is a major contributing factor to the unsustainable costs and delivery headaches that enterprises are currently battling.
In addition, general data management practices are unequipped to handle the current scope of data volumes in 2024. Across the enterprise organizations survey, 65% used 3 to 4 tools for data science tasks. Another 3-4 tools are used by 42% for data preparation and a further 46% with 4 tools for business intelligence.
However, the number of tools in use on average is a fundamental problem, according to SQream’s report. Applying several tools means there is often no single source of truth. It also stacks up the likelihood of workflow bottlenecks that slow the pace of innovation.
The survey pointed towards GPUs as a source of hope for both enterprises and the future of AI. 75% of those surveyed said that adding GPU instances to their analytics stack will have the most impact on their data analytics and AI and ML goals in 2024.
On this, Leff pointed out that “Leaders are increasingly recognizing the transformative power of GPU acceleration. The immense value of an order-of-magnitude performance leap is simply too valuable to be ignored in the race to become AI-driven.”
GPUs have the ability to accelerate the processing capabilities of the tech stack in use by an enterprise. This means that queries are delivered more quickly, making the system more cost-effective to run. Further, 65% of the leaders surveyed plan to prioritize optimizing their data pipelines. By doing so, the amount of time needed to prepare raw data will be reduced dramatically, saving resources in turn.
Why data management needs to evolve
While the promise of AI is indeed real, it’s taking longer to get there than expected.
For enterprises, a major part of the problem lies in the huge data pools that their operations generate combined with outdated data management practices equipped to handle the reality of modern big data projects.
The new report from SQream offers valuable insights into the challenges, along with realistic steps that can be taken to make tech stacks run more efficiently, cutting down on computing costs significantly.
With a new approach to data management, enterprise leaders will no longer need to compromise on AI projects due to steep running costs.
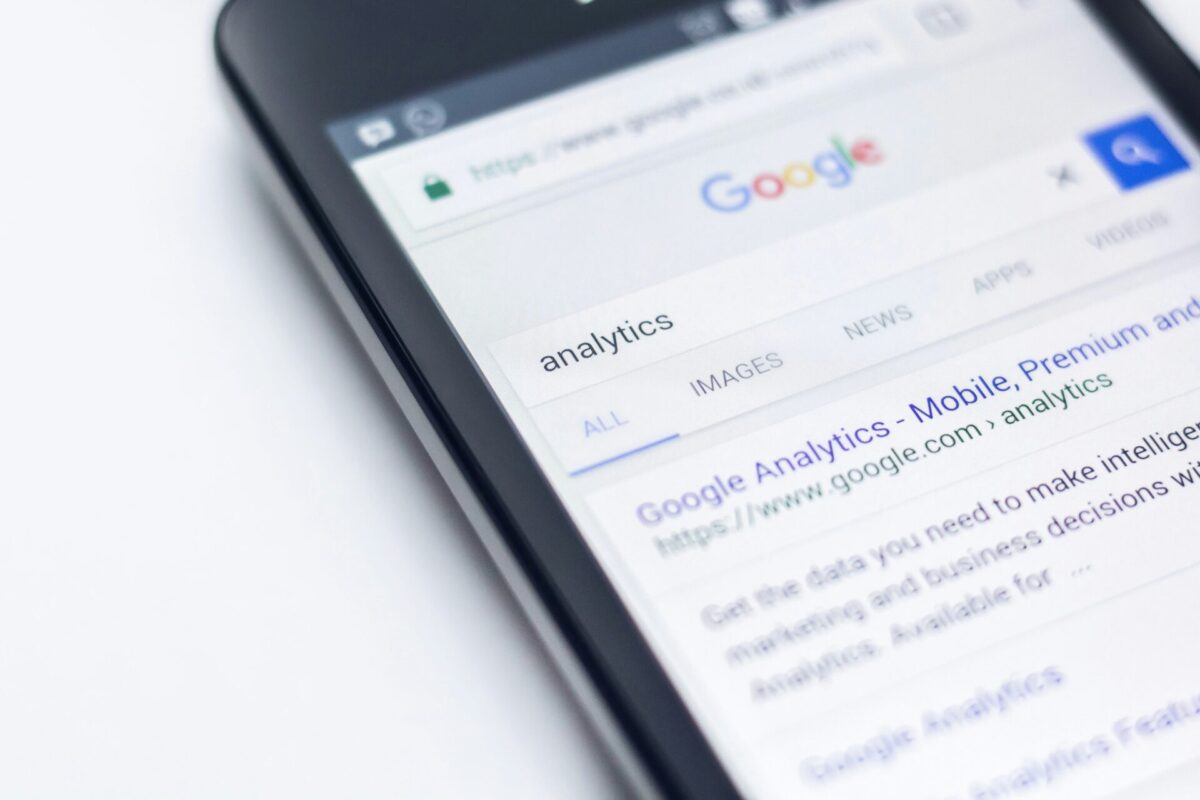
This article includes a client of an Espacio portfolio company